A new degree program at the University of Pennsylvania Stuart Weitzman School of Design is already beginning to bear fruit in the world. Deep Relief, a large-scale sculptural wall installation, was recently completed by students in the school’s Master of Science in Design: Robotic and Autonomous Systems (MSD-RAS) program. It is installed in the atrium of the Middletown Free Library outside Philadelphia, which held a dedication ceremony for the work on January 26.
The MSD-RAS program, now in its third year and led by assistant professor Robert Stuart-Smith, is a one-year, post-professional advanced architecture degree focused on the cutting edge of design and fabrication technologies. However, while much academic research remains confined to the lab and its simulated realm, this early cohort of designers happened into a unique opportunity to see their research realized. The original design for the atrium stair’s feature wall was cut during value engineering, leaving the library, designed by local practice Erdy McHenry, without a feature artwork. Principal Scott Erdy, who also serves as a lecturer at Weitzman and had helped design the robotics lab renovation for the MSD-RAS program, approached Associate Professor Andrew Saunders about repurposing the off-cuts of EPS foam blocks which were being robotically wire cut in the program’s introductory workshops. Instead, Saunders saw an opportunity to do something more ambitious.
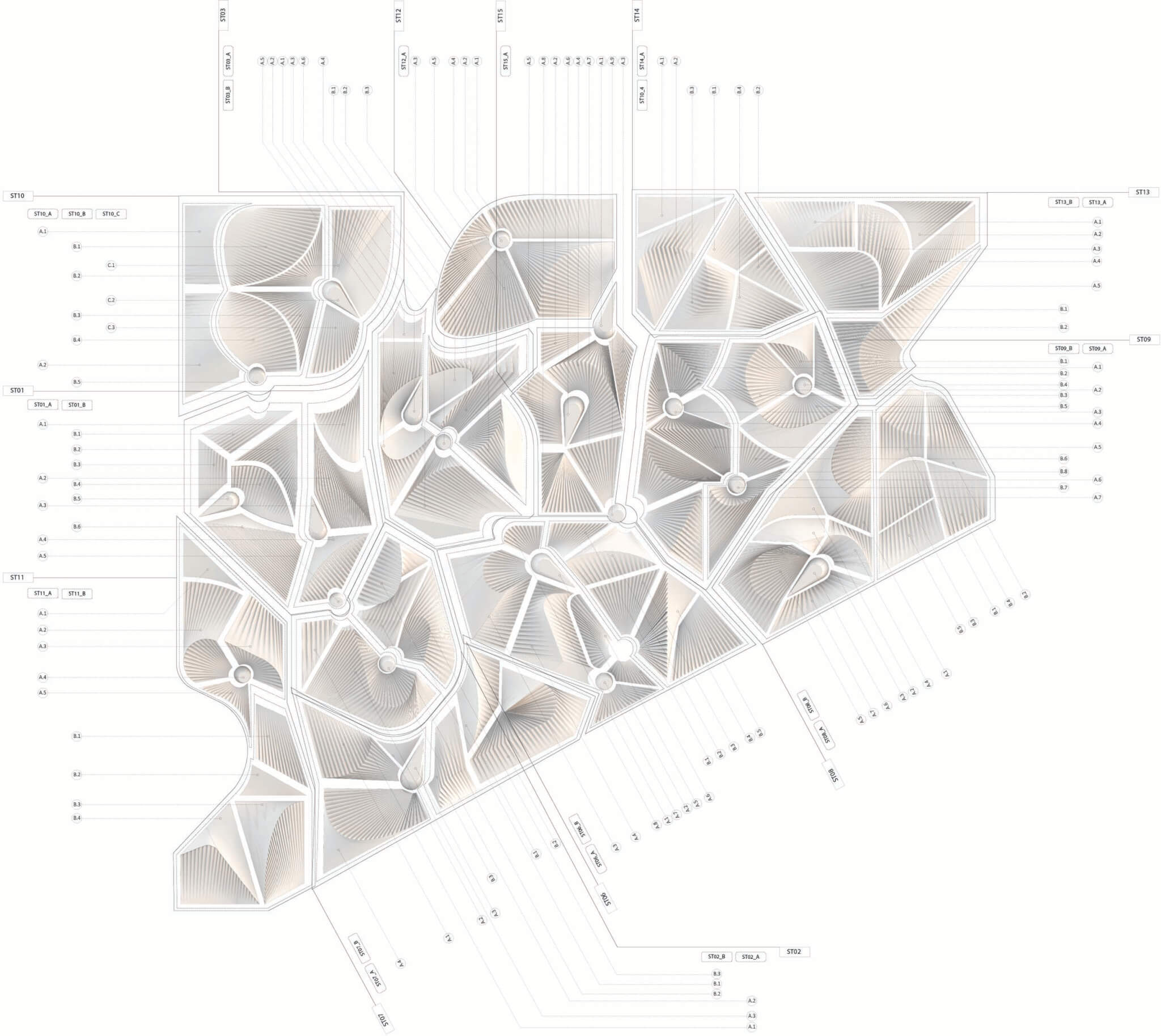
With institutional backing, the MSD-RAS program now had a specific design prompt and a (loose) deadline to guide their research. The result is an intricate composition of shadow and figural interplay as seen in a two-story, trapezoidal, 14-inch deep wall installation made of carved white EPS foam blocks.
Although robotic fabrication is far from commonplace in the contemporary construction industry, it is increasingly common for architecture schools to be equipped with advanced fabrication laboratories that boast such equipment. While describing any task a robotic arm can complete as “simple” is an exaggeration, hot-wire foam cutting is a relatively straightforward process. This expediency was part of the appeal for Saunders and the planners of the MSD-RAS program; in an accelerated program, time is of the essence. Seeing results quickly benefitted students as they learned, and the hot-wire cutting process was a way to accomplish this.

But the “A” in MSD-RAS stands for “autonomous,” not “automated”—the latter describes robotic fabrication processes that are ubiquitous in university settings, while the former are less so. To engage with the next generation of technology-focused design processes, “it’s not just about teaching them how to use the robot and make something,” Saunders told AN. “We need[ed] to bring in some notion of autonomy, so we started with the Convolutional Neural Network, or Style Transfer.” CNNs are a type of deep-learning, machine-vision algorithm that attempts to “see” and make distinctions in images similar to how human vision operates. The CNN is then used to redistribute and generate new iterations and images based upon features of the input training data. This work, which takes place within the subset of AI known as machine learning, began several years before Midjourney and other text-to-image tools made AI-produced imagery widely familiar.
The advantage of CNNs over more popular engines like Midjourney, for example, is the control over the training data and imagery that goes into the model in the first place: “We knew we had ruled surfaces, so we were only putting in ruled surfaces,” Saunders said of the custom training data the team developed. Those ruled surfaces—a surface formed by a straight line swept along two guiding curves—came from the inherent logic of the robotic hot-wire cutting arm, whose cuts are made using this geometry: The wire itself is the line, while the guiding curves are produced by the arm’s movement.
Though there are many famous designers that utilized ruled surfaces in architecture, such as Felix Candela’s thin-shell concrete structures, Saunders’s interest in complexity and relief led to a less-trod point of departure: the incredibly detailed ruled surfaces of Russian Constructivist sculptors Naum Gabo and Antoine Pevsner. Designers modeled and developed training data that learned from the logic of these sculptors, which embedded a deep knowledge of fabrication and three-dimensional geometry within the CNN. The CNN, after learning these precedents, redistributed them into new patterns, compositions, and types of cuts.
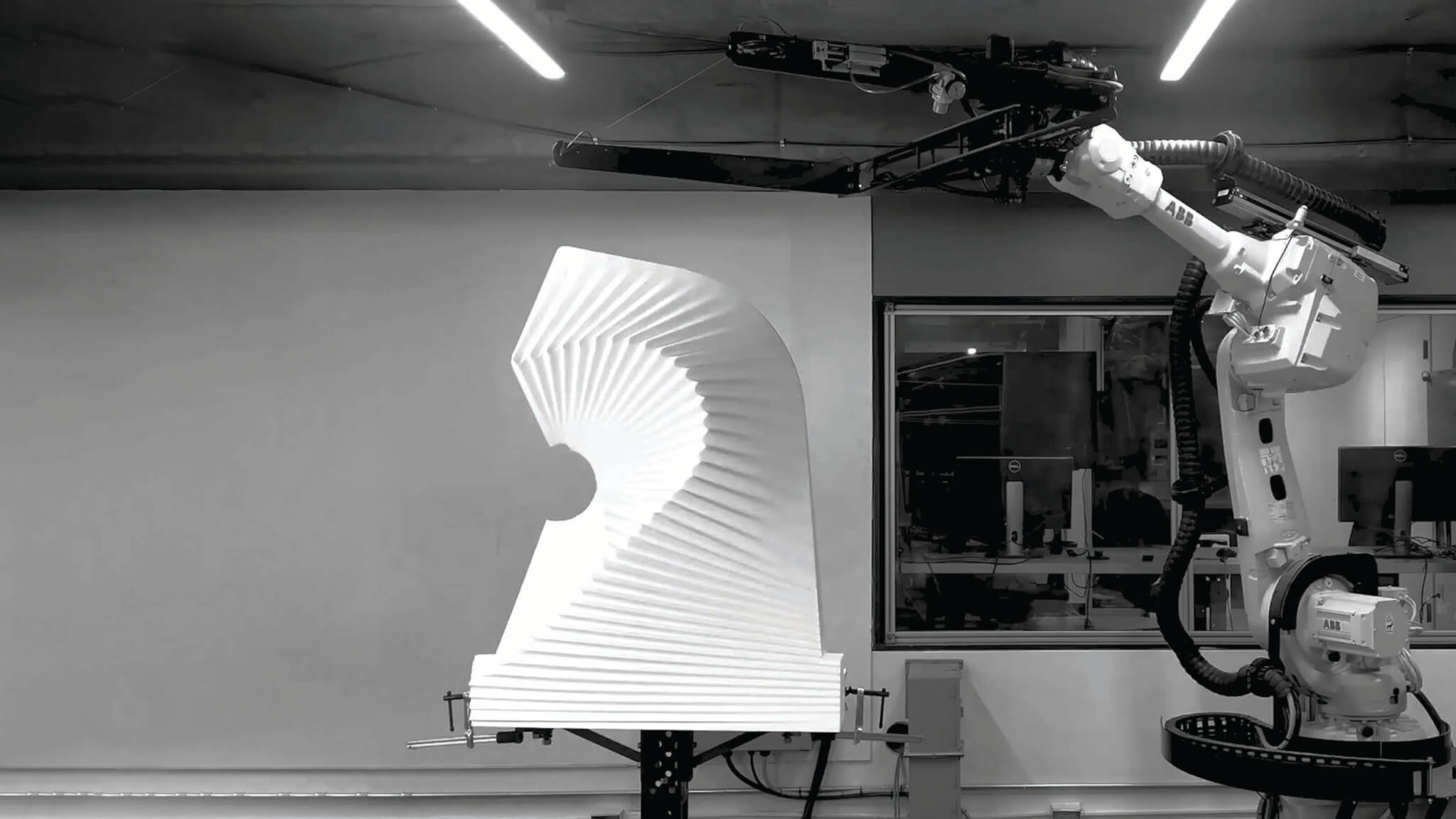
The result is still a raster two-dimensional image that requires interpretation into a three-dimensional digital model that fabrication data can be generated from via Grasshopper scripting. For Saunders, this interpretation is endemic to the architectural process, regardless of the technology at play: “It’s a very manual or explicit modeling process [to go from CNN to 3D].” The knowledge of the types of cuts embedded in the training data by the researchers can be surveyed in the new CNN-generated images; in doing so, the designers have learned how to create but also how to read and translate, and a deeper knowledge with unexpected or unintended consequences occurs. While the project is a bit radical—Saunders doesn’t know of another permanent built project of this scale which has utilized AI into a three-dimensional object—it’s also just fundamental stuff: “At some level its basic architecture 101, looking at 2D images that have spatial implications and asking how to make them 3D.”

Finally culminating in a large-scale work after two years of research, fundraising, design, prototyping, and fabrication of pieces in the off-hours of Meyerson Hall’s robotic lab, Deep Relief snapped into place on-site much faster than anticipated, especially for an installation made of irregular, custom-fabricated units. Despite the number of unique pieces, the assembly is relatively standard: Each piece of foam, finished with epoxy and paint, has an MDF backing and is hung on the wall using Z-clips. The team allotted four days for installation, but this was overkill. Armed with fabrication drawings and a 3D-printed resin scale model with labeled pieces, it only took workers half a day to complete. “[The installation process] was literally perfect,” Saunders lauded, as no misfits were reported, and no pieces had to be modified or replaced.
Deep Relief is a proof of concept for AI-driven design processes, robotic wire-cutting, and the MSD-RAS program itself. It’s also a strong testament to the benefit of advanced digital fabrication methods. While there was a somewhat lengthy two-year period before the project was eventually installed, that timeline for proprietary experimentation to be implemented in the world is speedy relative to most contemporary scholastic research. Further, the success and ease of installation is a counterargument to the idea that conventional construction means and methods are easier or more reliable than newer and novel processes. The precision and tight tolerances that automated fabrication technologies offer are unrivaled relative to manual methods; any additional upfront costs associated with the unknown may well be offset by elimination of rejected rework.

Deep Relief’s duality is remarkable, as it is both a permanent work and a model. Now, of course, its qualities and characteristics are a benefit to Middletown bookworms and makerspace aficionados alike. But it also acts as a model, in multiple senses. It’s a model for the MSD-RAS program and digital fabrication broadly. (Saunders is already working on how the concepts and research explored in this project could translate to issues of enclosure and structure at the scale of a full building enclosure.) Because of its scale, materiality, color, and complex angular geometries, it also reads as an architectural model which portends subsequent constructions, much like typical models precede the buildings they describe. The piece can be read as singular thing but also as a series of objects that nest perfectly together—the library wall becomes gallery space, a suggestion of possibility which can’t be exhausted by the experience of the work’s physical instantiation. “For me,” said Saunders, “I think the project is interesting as an answer—not the answer—for how to go 3D [from AI] and what the architectural consequences are.”
Davis Richardson is an architect at REX and has taught at NJIT and the Architectural Association.
Project credits:
- Research and Design Director: Associate Professor Andrew Saunders
- Robotic Research Assistants: Penn Praxis Fellow Riley Studebaker, Penn Praxis Fellow Mathew White, Claire Eileen Moriarty
- Research Assistants: Penn Praxis Fellow Caleb Ehly, Penn Praxis Fellow Benjamin Hergert, Yujie Li, Jesse Allen, Macarena De La Piedra, Cecily Nishimura
- Materials and Construction: Universal Foam, L.J. Paollela Construction